Nowadays we see the use of Artificial Intelligence (AI) in every field of study, even in image processing, data analytics, text processing, robotics, industries, software technologies, etc. Day by day increasing the use of AI helps users to perform automated tasks without involving the human physically present. The work can be completed automatically within the specified time as per the requirement. Even in the field of Software Engineering, the use of AI is growing day by day to perform automated tasks without causing errors. AI in software engineering provides automated non-error calculations, in the field of software engineering it provides the automated testing of software, automatic debugging of software, etc. to provide high quality (quality assurance), and efficiency of software applications within the budget.
Software Engineering is the method of developing, testing, and deploying computer software to solve problems and issues in the real world by utilizing software principles and best practices. It provides an organized and professional approach from developing the software to the deployment of the software. In the field of software engineering software metrics play a very important role. The software metrics are used to evaluate the reusability, quality, portability, functionality, and understandability. The AI-based software metrics are error-free and automated, they will used to identify or predict the defects in the software and efficient solutions in the real world scenario without involving humans.
Background
Many researchers describe different software metrics and provide efficient solutions for the software metrics. Some research also describes automated solutions using deep learning techniques to improve the software metrics. Without using Artificial intelligence, some chances degrade the quality of the final software products. It may also include a lack of functionality and require more human interactions due to which it increases the cost of the software. Some researchers describe deep learning-based techniques to solve software metrics problems and provide efficient results. However, the can be a lack in using the dataset and training of the data. Using the correct dataset may cause erroneous training of data and provide the wrong results. The wrong results may cause issues and degrade the quality of the software metrics. Therefore, this blog provides the proposed solution that aims to solve the problem of software metrics using Artificial Intelligence.
Basic Concept
According to the authors, there are several studies considered and provided many definitions. Therefore, before discussing the proposed approach let us discuss some basics about the software metrics as discussed.
As discussed above the software metrics are used to evaluate the reusability, quality, portability, functionality, and understandability of the software to achieve high-quality software. The software, metrics are of two types: the system-level metrics and component-level metrics as described in Figure 1.
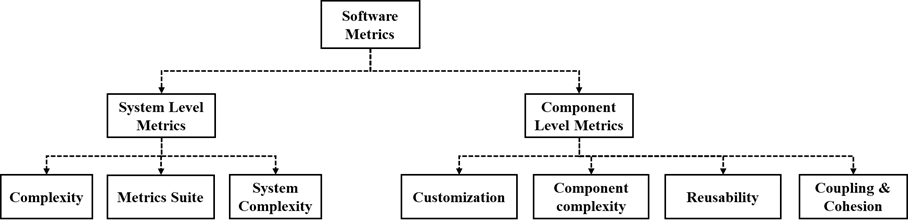
Figure 1: Software Metrics Categories
- System Level Metrics: The system level metrics are further divided into three types that is Complexity, Metrics Suite, and System Complexity.
- Component level Metrics: The component level metrics are further divided into four types that are Customization, component complexity, reusability, and coupling and cohesion metrics.
- Complexity Metrics: The complexity metrics are the type of system-level metrics. There are many definitions given by many authors. According to IEEE, complexity is defined as the quality where the component or any system design and implementation is complex to understand and authenticate.
- Metric Suite: It provides the requirements and functionality of the software that is needed by the users. It ensures to provide users a high quality, satisfactory fault-free software products.
- System Complexity Metric: It is defined as the component metric having a set of components in the system.
- Customization: The customization metric identifies whether the component can be customized according to the user’s needs or not.
- Component Complexity Metrics: The Component Complexity Metrics have component plain complexity (CPC), Component Static Complexity (CSC), and Component Dynamic Complexity (CDC).
- Component Reusability Metric: This type of metric is the ratio of the sum of interface methods that provides common reuse component features used in a feature.
- Coupling & Cohesion metrics: It is the degree or power with which software components are related to each other.
Proposed Approach
The proposed approach describes the AI-based method to identify as well as predict the defects in the software metrics. In this proposed approach, first, the real-time software metrics dataset is collected from multiple sources. The software metrics dataset may include the data regarding the identified software metrics with labels. The Figure 2 describes the architecture of the proposed approach and systematic details process of this approach.
Figure 2: Proposed Approach
- Obtaining data: This step is very essential and very important step of the approach. Correct data means high performance with minimum defects and results in high-quality software. Therefore, in this step, the labeled dataset of the software metrics will be collected. The dataset will include above 50K software modules and the number of defects predicted in the software module. The predicted defects can be binary values in the form of zero and one.
- Data Pre-Processing: After data, collection data pre-processing is an essential step of this proposed approach. After data collection, the data must be cleaned and normalized properly to make the analysis simpler and more efficient. In this step, the data is cleaned by removing empty rows, duplicate values, indexing of data, etc.
- Artificial Intelligence Model: After data, cleaning the Artificial Intelligence Model will be applied that analyzes the data such as determining the combinations, and automating the detection procedure without human interaction. AI model can be any algorithm applied such as Linear Regression, Logistic Regression, Naïve Bayes, Support Vector Machine, etc. These algorithms will analyze the data and determine the combinations. In this step, the data can also be converted into some kind of vectors also known as numbers based on the software metrics given in the dataset. The AI model is also known as the machine-learning model.
- Training and Testing: After the AI model is applied, the data will be split into train sets and test sets that are different from each other. 75% data is given for the train set and the remaining 25% of the data is given for the test set. Then the model is trained on the train set that will train the model based on the combinations and the test set will be used for our model that does not contain any labels, the machine will identify the faults automatically based on the training of the model.
- Results: After applying the AI or ML model on the test set the results are obtained on the test set that will determine how best the model works. The results will be determined in terms of accuracy, the area under the curve, f1-score, confusion matrix, etc. The expected accuracy will achieve 98% by applying logistic regression on the test set.
This blog is inspired and in contributed with Dr. Kiran Narang, Department of Computer Science Engineering, SRM University
REFERENCES
[1]. https://www.techtarget.com/whatis/definition/software-engineering
[2]. https://ieeexplore.ieee.org/document/8443016
[3]. https://en.wikipedia.org/wiki/Software_metric
[4]. https://www.sciencedirect.com/science/article/abs/pii/S0925231219316698
[5]. https://www.mdpi.com/2227-7390/10/17/3120
[6]. https://www.sciencedirect.com/science/article/pii/S0164121222002138
[7]. https://viso.ai/deep-learning/ml-ai-models/