Alexa is a cloud-based software program that acts as a voice-controlled virtual personal assistant. Alexa works by listening for voice commands, translating them into text, interpreting the text to carry out corresponding functions, and delivering results in the form of audio, video, or device/accessory triggers.
Hidden Markov Models (HMMs) are a type of probability model that can be used in Natural Language Understanding (NLU) to help programs come to the most likely decision based on both previous decisions and observations.
Machine learning plays a critical role in improving Alexa’s ability to understand and respond to voice commands over time.
Alexa has three main parts: Wake word, Invocation name, and Utterance. Here is a breakdown of each part:
- Wake word: This is the word that users say to activate Alexa. By default, the wake word is “Alexa,” but users can change it to “Echo,” “Amazon,” or “Computer.
- Invocation name: This is the unique name that identifies a custom skill. Users can invoke a custom skill by saying the wake word followed by the invocation name. The invocation name must not contain the wake words “Alexa,” “Amazon,” “Echo,” or the words “skill” or “app.
- Utterance: This is the spoken phrase that users say to interact with Alexa. Users can include additional words around their utterances, and Alexa will try to understand the intent behind the words.
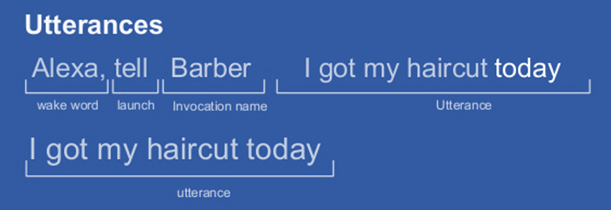
What is NLP?
Natural Language Processing (NLP) is a key component of Alexa’s functionality. NLP is a branch of computer science that involves the analysis of human language in speech and text. It is the technology that allows machines to understand and interact with human speech, but is not limited to voice interactions. NLP is the reader that takes the language created by Natural Language Generation (NLG) and consumes it. Advances in NLP technology have allowed dramatic growth in intelligent personal assistants such as Alexa.
Alexa uses NLP to process requests or commands through a machine learning technique. When a user speaks to Alexa, the audio is sent to Amazon’s servers to be analysed more efficiently. To convert the audio into text, Alexa analyses characteristics of the user’s speech such as frequency and pitch to give feature values. The Alexa Voice Service then processes the response and identifies the user’s intent, making a web service request to a third-party server if needed.
In summary, NLP is the technology that allows Alexa to understand and interact with human speech. It is used to process requests or commands through a machine learning technique, and NLU is a key component of Alexa’s functionality that allows it to infer what a user is asking for when they ask a question in a variety of ways.
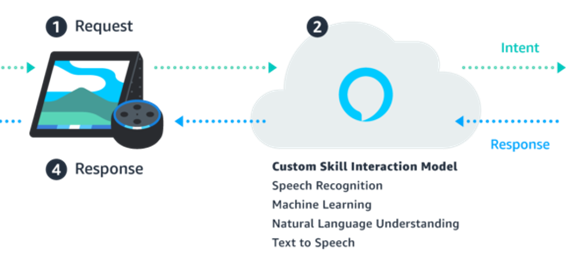
Hidden Markov Model (NLU Example)
HMMs are used in Alexa’s NLU to help understand the meaning behind the words spoken by the user. Here is an example of how HMMs can be used in Alexa’s NLU:
- The user says “Alexa, play some music.”
- The audio is sent to Amazon’s servers to be analyzed more efficiently.
- The audio is converted into text using speech-to-text conversion.
- The text is analyzed using an HMM to determine the user’s intent. The HMM takes into account the previous decisions made by the user, such as previous music requests, as well as the current observation, which is the user’s request to play music.
- Alexa identifies the user’s intent as “play music” and performs the requested action.
Conclusion
In summary, Alexa’s NLP architecture involves converting the user’s spoken words into text, processing the text to identify the user’s intent, and performing complex operations such NLU using the Alexa Voice Service.