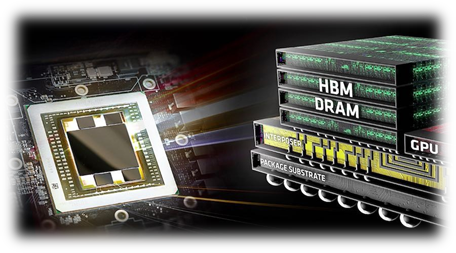
High Bandwidth Memory 3 (HBM3): Overcoming Memory Bottlenecks in AI Accelerators
With the rise of generative AI models that can produce original text, picture, video, and audio material, artificial intelligence (AI) has made major strides in recent years. These models, like large language models (LLMs), were trained on enormous quantities of data and need a lot of processing power to function properly. However, because of their high cost and processing requirements, AI accelerators now require more effective memory solutions. High Bandwidth Memory, a memory standard that has various benefits over earlier memory technologies, is one such approach.
How HBM is relevant to AI accelerators?
Constant memory constraints have grown problematic in a number of fields over the past few decades, including embedded technology, artificial intelligence, and the quick growth of generative AI. Since external memory interfaces have such a high demand for bandwidth, several programs have had trouble keeping up. An ASIC (application-specific integrated circuit) often connects with external memory, frequently DDR memory, through a printed circuit board with constrained interface capabilities. The interface with four channels only offers about 60 MB/s of bandwidth even with DDR4 memory. While DDR5 memory has improved in this area, the improvement in bandwidth is still just marginal and cannot keep up with the continuously expanding application needs.
However, a shorter link, more channels, and higher memory bandwidth become practical when we take the possibility of high memory bandwidth solutions into account. This makes it possible to have more stacks on each PCB, which would greatly enhance bandwidth. Significant advancements in high memory bandwidth have been made to suit the demands of many applications, notably those demanding complex AI and machine learning models.
The latest generation of High Bandwidth Memory
The most recent high bandwidth memory standard is HBM3, which is a memory specification for 3D stacked SDRAM that was made available by JEDEC in January 2022. With support for greater densities, faster operation, more banks, enhanced reliability, availability, and serviceability (RAS) features, a lower power interface, and a redesigned clocking architecture, it provides substantial advancements over the previous HBM2E standard (JESD235D).
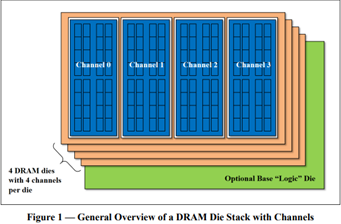
[Source: HBM3 Standard [JEDEC JESD238A] Page 16 of 270]
P.S. You can refer to HBM3 Standard [JEDEC JESD238A]: https://www.jedec.org/sites/default/files/docs/JESD238A.pdf for further studies.
How does HBM3 address memory bottlenecks in AI accelerators?
HBM3 is intended to offer great bandwidth while consuming little energy, making it perfect for AI tasks that need quick and effective data access. HBM3 has a number of significant enhancements over earlier memory standards, including:
Increased bandwidth
Since HBM3 has a substantially larger bandwidth than its forerunners, data may be sent between the memory and the GPU or CPU more quickly. For AI tasks that require processing massive volumes of data in real time, this additional bandwidth is essential.
Lower power consumption
Since HBM3 is intended to be more power-efficient than earlier memory technologies, it will enable AI accelerators to use less energy overall. This is crucial because it may result in considerable cost savings and environmental advantages for data centers that host large-scale AI hardware.
Higher memory capacity
Greater memory capacities supported by HBM3 enable AI accelerators to store and analyze more data concurrently. This is crucial for difficult AI jobs that need access to a lot of data, such as computer vision or natural language processing.
Improved thermal performance
AI accelerators are less likely to overheat because to elements in the architecture of HBM3 that aid in heat dissipation. Particularly during demanding AI workloads, this is essential for preserving the system’s performance and dependability.
Compatibility with existing systems
Manufacturers of AI accelerators will find it simpler to implement the new technology because HBM3 is designed to be backward-compatible with earlier HBM iterations without making substantial changes to their current systems. This guarantees an easy switch to HBM3 and makes it possible for quicker integration into the AI ecosystem.
In a word, HBM3 offers enhanced bandwidth, reduced power consumption, better memory capacity, improved thermal performance, and compatibility with current systems, making it a suitable memory choice for AI accelerators. HBM3 will play a significant role in overcoming memory constraints and allowing more effective and potent AI systems as AI workloads continue to increase in complexity and size.
Intellectual property trends for HBM3 in AI Accelerators
HBM3 in AI Accelerators is witnessing rapid growth in patent filing trends across the globe. Over the past few years, the number of patent applications almost getting doubled every two years.
MICRON is a dominant player in the market with 50% patents. It now holds twice as many patents as Samsung and SK Hynix combined. Performance, capacity, and power efficiency in today’s AI data centers are three areas where Micron’s HBM3 Gen2 “breaks new records.” It is obvious that the goal is to enable faster infrastructure utilization for AI inference, lower training periods for big language models like GPT-4, and better total cost of ownership (TCO).
Other key players who have filed for patents in High bandwidth memory technology with are Intel, Qualcomm, Fujitsu etc.
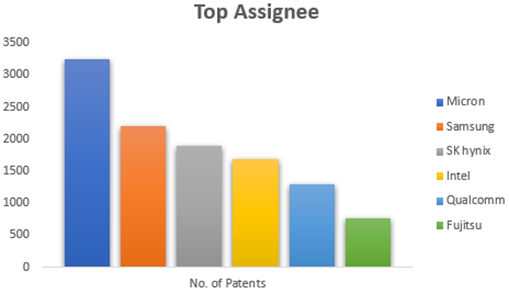
[Source: https://www.lens.org/lens/search/patent/list?q=stacked%20memory%20%2B%20artificial%20intelligence]
Following are the trends of publication and their legal status over time:
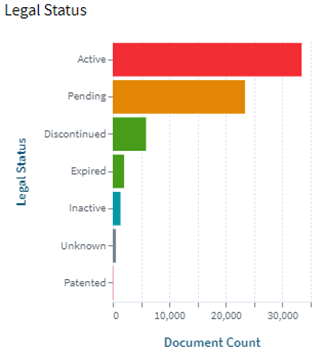
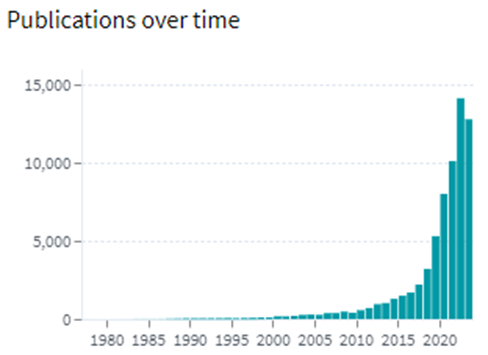
[Source: https://www.lens.org/lens/search/patent/list?q=stacked%20memory%20%2B%20artificial%20intelligence]
These Top companies own around 60% of total patents related to UFS. The below diagram shows these companies have built strong IPMoats in US jurisdiction.
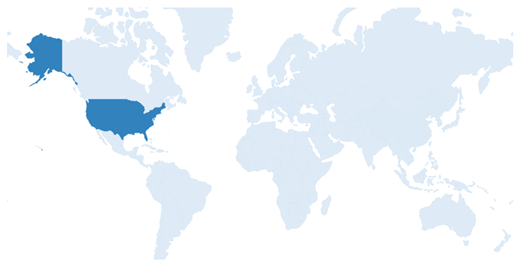
[Source: https://www.lens.org/lens/search/patent/list?q=stacked%20memory%20%2B%20artificial%20intelligence]
Conclusion
In summary, compared to earlier memory standards, HBM3 provides larger storage capacity, better bandwidth, reduced power consumption, and improved signal integrity. HBM3 is essential for overcoming memory limitations in the context of AI accelerators and allowing more effective and high-performance AI applications. HBM3 will probably become a typical component in the next AI accelerator designs as the need for AI and ML continues to rise, spurring even more improvements in AI technology.
Meta Data
The performance of AI accelerators will be improved by the cutting-edge memory technology HBM3, which provides unparalleled data speed and efficiency.