What is Kubernetes?
Kubernetes (K8s) is an open-source platform that facilitates the execution of containerized applications in a virtual environment via Application Program Interfaces (APIs). Containerized applications are programs that are executed in containers. Containers are the virtual entities that hold the primary code for the execution of an application, its dependencies of that application and the configuration files of that application. Containerized applications are widely adapted because they facilitate the execution of multiple applications in a single host by isolating them from the core Operating System. This makes Kubernetes a go-to for users/developers to test, assess, and deploy their applications.
Kubernetes Architecture
Kubernetes employs a Master-Slave architecture. Kubernetes Cluster is divided into two separate planes:
i. Control Plane: Also known as the Master Node, the Control plane can be interpreted as the brains of Kubernetes. It is the policy maker that applications executed in Kubernetes clusters have to follow. It consists of:
a. API server: The API server is the entity that authenticates and authorizes a developer and allows interaction between the developer and Kubernetes Cluster. The API server configures and manipulates entities in the data plane via Kubernetes Controller-Manager, Kubernetes Scheduler, and Key-Value Store (Etcd).
b. Kubernetes Controller-Manager: It is the entity in the Control Plane that is responsible for keeping the system in a desired state, as per the instructions obtained from the API server. It constantly monitors the containers, Pods, and Nodes and tweaks them to bring them to the desired state.
c. Kubernetes Scheduler: It is the entity in the Control plane responsible for deploying applications in Worker Nodes received through the API server. It schedules the applications as per their requirements of resources, like memory, identifies suitable Pods, and places them in suitable Worker Nodes in the Kubernetes Clusters.
d. Key-Value Store (Etcd): It is a storage that can be placed within the control plane or independent of it. Key-value Store, as the name suggests, stores all the data of the Kubernetes Cluster, i.e., it provides a restore point to the whole of the Kubernetes Cluster.
ii. Data Plane: The Data Plane is a cluster of Kubernetes Worker Nodes that executes the policies made by the Control plane for the smooth operation of applications within the Kubernetes Cluster. Worker nodes are the machines that run containerized applications and provide the necessary resources for the applications to run smoothly. Each Worker Node consists of:
a. Kubelet: Kubelet is the entity within the Worker Node that is responsible for connecting that node with the API server in the Control Plane and reporting the status of Pods and containers within the node. This facilitates the resources assigned to that node to become a part of the Kubernetes Cluster. It is also responsible for the execution of works received from the API server to keep the node in a desired state by making the necessary changes as per API server instructions.
b. Kube-proxy: It is responsible for routing traffic from the users through the Internet to the correct applications within a node by creating/altering traffic routing policies for that node.
c. Pods: Pods are the entities in the Worker Node that have containers within them. Although it is possible to host multiple application instances in a Pod, running one application instance in one Pod is recommended. Pods are capable of horizontal scaling, i.e., they are created according to the application instance needs. If assigned node resources are available, Pods can utilize more resources than assigned to them- if needed. Pods, along with containers, are capable of running on multiple machines. The resources of the Pods are shared among the containers it hosts.
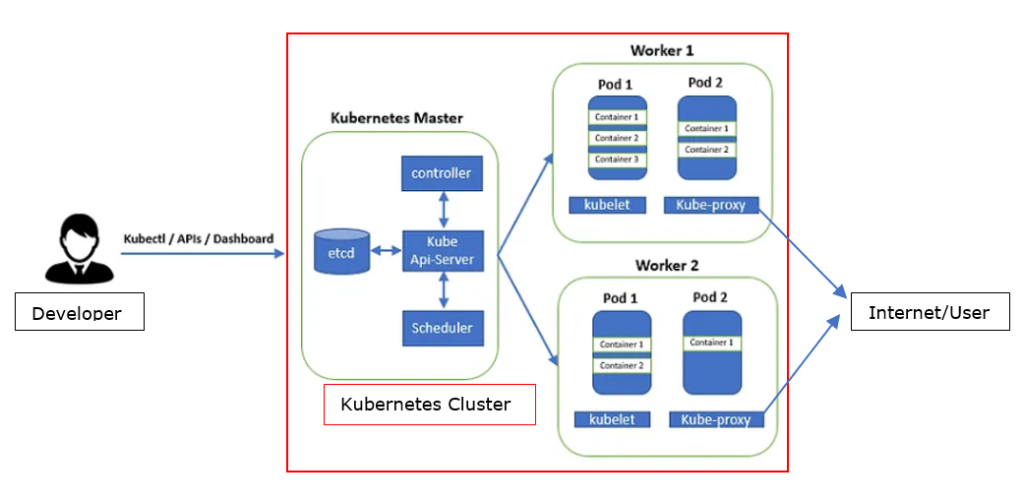
HBM Layout (Source: Medium)
Deploying an Application in Kubernetes:
i. The developer should have a Service account. This account is needed to authenticate and authorize a developer. Also, this service account is used for authentication against the API server when the application needs access to protected resources.
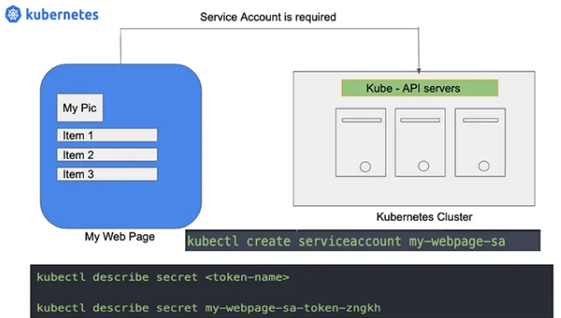
Service Account Requirement (Source: Medium)
ii. Create a new Node or select an existing node according to the application requirement (memory, RAM, etc).
iii. The intended application should be packed in a Docker image or similar container format. A Docker image is a software package that has all the necessary programs, dependencies, runtimes, libraries, and configuration files for an application to run smoothly.
iv. The developer should define Kubernetes Manifest as a YAML or JASON file. The Kubernetes Manifest defines the desired state for the application to be deployed. It consists of:
a. Configmaps: As the name suggests, Configmaps have configuration data of the application to be deployed. It has supporting configurations, like environment variables for the intended application. The total size of this data is less than 1MB.
b. Secrets: Kubernetes secrets are similar to Configmaps, but hold secure information. They hold supporting files, like passwords, for the application that is to be deployed.
c. Deployments: Deployments define the procedure of creating and updating application instances for the application to be deployed.
d. Kubernetes Service: It is the entity that assigns an IP address or hostname to the application that is to be deployed. When the assigned name is matched to a user’s search string, the application is presented to the user through the internet via Kube Proxy.
v. The developer places the Docker image through the Kubernetes API server. The API server pulls the Docker image to create the containers in the Pods, to deploy the intended application.
vi. Once the intended application is deployed in the pods, the developer can monitor, update, change, and edit the application as per the requirement through Kubectl from the developers’ service account through the API server in the control panel.
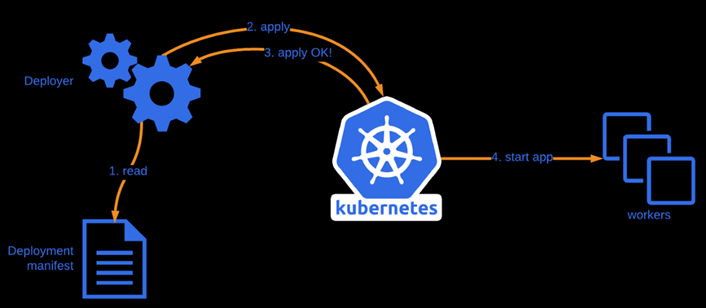
Deployment Flow (Source: Polarsquad)